Promoted by the evolution of artificial intelligence and deep learning, more and more intelligent applications have emerged on mobile devices. As one of the most representative deep learning technologies, deep neural networks (DNNs) have been widely applied to many fields such as computer vision, speech recognition, natural language processing, and audio recognition, where they have produced results comparable to and in some cases surpassing human expert performance. However, the heavy computation, memory, and energy demands of the deep learning model restrict their deployment on resource-constrained mobile devices.
The MIC Lab at SFSU performs research in efficient mobile computing, deep learning acceleration, and intelligent application development. A key focus of MIC Lab is to achieve computational acceleration for deep learning on low-power hardware without heavy compute or storage capabilities. Our research addresses the efficiency, reliability, and security challenges in the algorithm design and system optimization as well as application development to create intelligent mobile edge computing system.
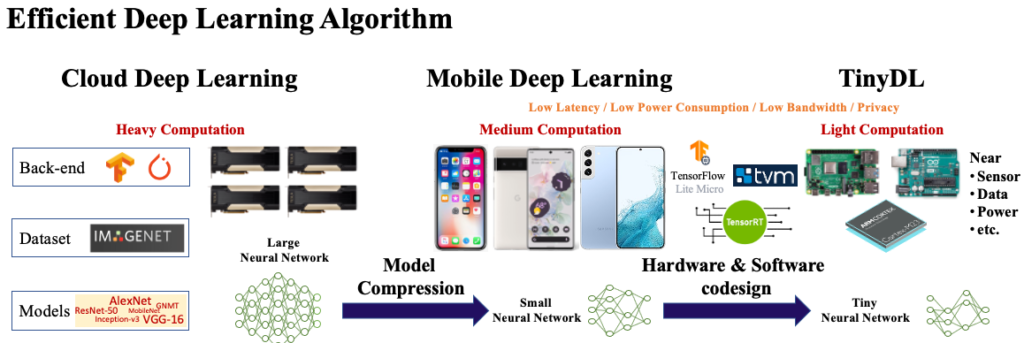
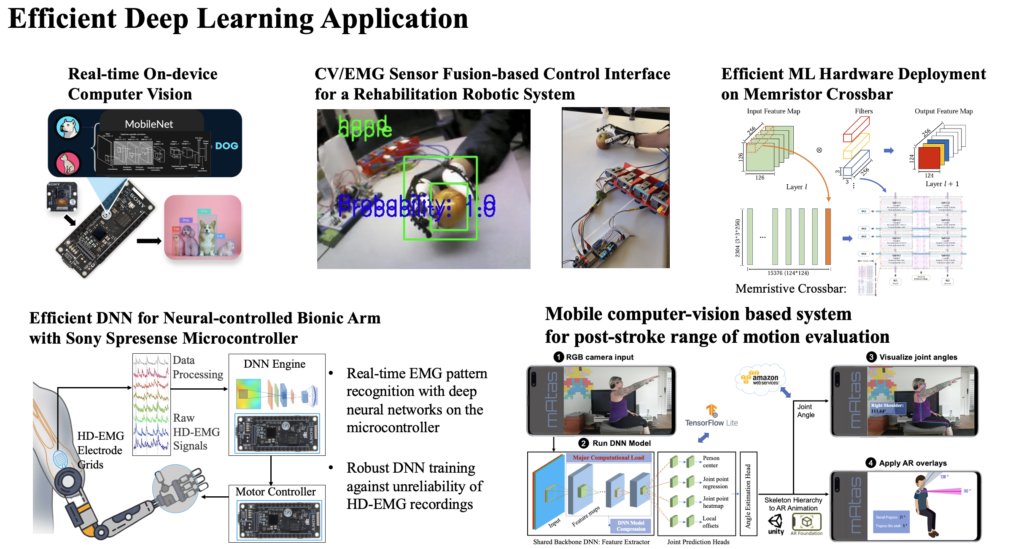